Artificial intelligence (AI) provides industrial maintenance teams with a powerful opportunity to streamline operations and optimize their maintenance programs. According to the latest American Manufacturing Resurgence survey by Forbes and Xometry, 80% of manufacturing CEOs plan to invest in AI over the next two years, while 61% of CEOs are “actively embracing AI [already].”
While implementing AI might seem daunting, maintenance teams slow to adopt AI risk leaving themselves vulnerable to market and labor conditions and falling behind their competitors. In this post, we’ll explore five ways AI can optimize your maintenance strategy, transforming it from a costly necessity to a proactive, cost-effective program.
How Can You Use Artificial Intelligence to Improve Your Maintenance Strategy?
Here are five ways AI can improve your maintenance strategy.
1. AI-Powered Predictive Maintenance
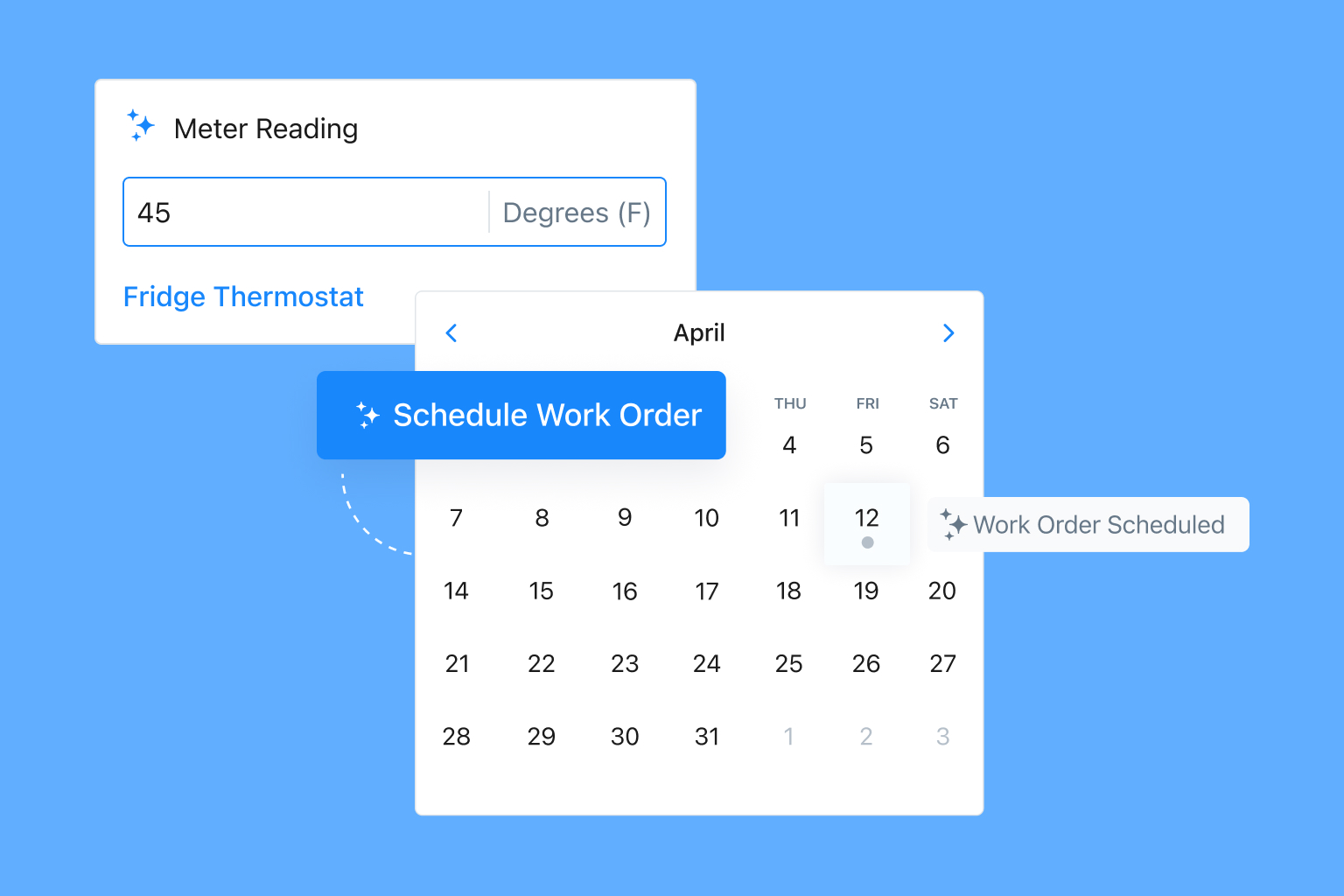
Predictive maintenance is a preventive maintenance approach that uses sensor technology and data analytics to predict breakdowns and support dynamic maintenance planning. A predictive maintenance strategy helps teams focus their maintenance activities on the equipment and assets at high risk of breakdowns and avoid unnecessary maintenance costs.
Predictive maintenance relies heavily on data. First, technicians fit their equipment with Industrial Internet of Things (IIoT) monitoring instruments, which collect data about vibrations, temperature, pressure, and other equipment condition information. Maintenance teams then analyze this data to identify patterns or trends indicating potential equipment failures and create dynamic maintenance schedules. For example, an analysis of historical maintenance data may show that an increase in an asset’s vibration levels above a certain threshold will lead to failure. Using this valuable insight, the maintenance team can then monitor real-time data to identify maintenance needs based on the condition of the asset and schedule maintenance work as needed.
AI and machine learning algorithms improve the accuracy of the predictive models that power predictive maintenance programs by dramatically increasing the volume of data analyzed and the speed of data processing. The more data the AI algorithms analyze, the more accurate your predictive analytics will become; AI can solve ten problems in one minute, while the human mind can solve only a single problem in five minutes. With better and more accurate predictions, maintenance teams can increase operational efficiency by minimizing redundant work, reduce unplanned downtime by preventing equipment failures, and drive cost savings through optimizations.
2. AI-Driven Anomaly Detection
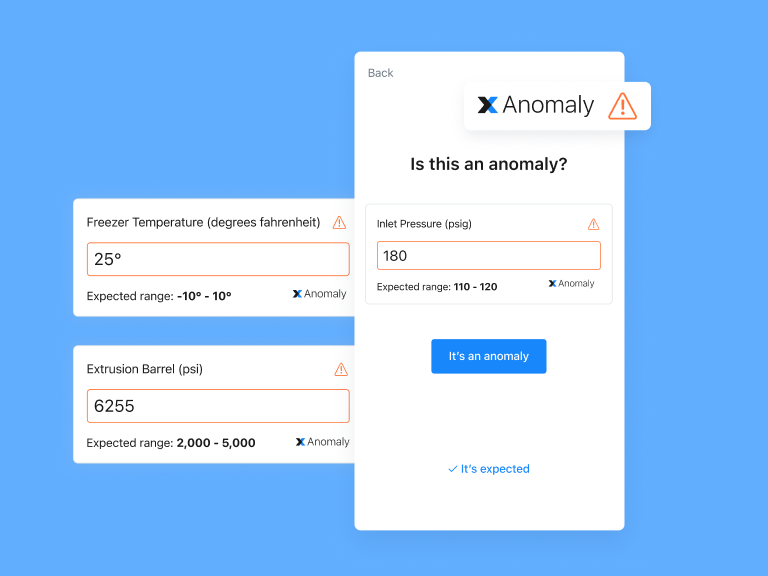
Maintenance strategies that rely on data, like condition-based or predictive maintenance, are only as effective as the quality of the data set they use. Inaccuracies within the data set can jeopardize the effectiveness of these approaches, as the errors can lead to incorrect recommendations and predictions that can throw off your team’s entire maintenance schedule. Because maintenance teams often collect asset data manually and don’t always have the time or access to compare current readings with historical data, there’s an increased risk of data inconsistencies and inaccuracies.
AI-driven anomaly detection can provide you with the necessary guardrails to protect the quality and integrity of your data set. AI models trained on historical data can automatically flag abnormal meter readings or records and alert technicians to double-check before finalizing the data input. With anomaly detection, maintenance teams can reduce inconsistencies and errors in their data sets, improving the quality and output of the AI models.
3. Automated Inventory Management

Effective inventory management is one of the most crucial elements of any maintenance strategy. Imagine, for example, scheduling maintenance tasks only to find that technicians can’t complete them because they lack the correct tools or spare parts. With supply chain disruptions and the rising cost of materials, purchasing the right tools and spare parts can be difficult and costly. While stocking up may seem like an obvious solution to unplanned stockouts, it comes with its own challenges: expensive carrying and inventory costs that increase operational expenses and cut into profitability.
AI systems can analyze historical and real-time equipment, inventory, and purchasing data to help maintenance teams maintain optimal inventory levels. These systems can identify patterns in your parts usage and purchase history to recommend when you should restock parts. When configured properly, AI systems can make purchases for your team to maintain optimal inventory levels and automate the inventory management process.
4. AI-Generated Standard Operating Procedures

Successful and consistent maintenance work relies on standardization, ensuring that technicians complete the work correctly every single time. Maintenance teams typically use standard operating procedures (SOPs)—step-by-step, repeatable processes for routine tasks that act as documentation for technicians—to establish standardized guidelines for completing each maintenance task and ensure the consistency and quality of the work.
However, given the sheer number and variety of maintenance tasks required to keep a facility up and running, creating detailed SOPs from scratch for each process can be challenging. Using generic SOP templates and adapting them to meet your needs can help maintenance teams speed up the work. However, this process still requires manual configuration, the labor of which can add up if you have hundreds or thousands of procedures and processes.
With generative artificial intelligence (GenAI), you can automatically create tailored SOPs by supplying the underlying large language models (LLMs) with details about your facilities, equipment, and processes. Once trained, these GenAI models can create required procedures with a simple prompt, request, or uploaded document, like PDFs, manuals, and images.
While these AI-generated procedures may still require tweaks and adjustments, they provide you with a valuable head start, substantially cutting down the time spent on manual procedure creation or customization of generic templates.
5. Advanced Voice Transcriptions

Manually typing or handwriting data to fill out required fields is a restrictive method of communication for frontline workers. When you are on the shop floor responding to incidents, it’s not always easy to find the time and space to fill in the required information manually. It slows communication and reduces the level of contextual data in each work order.
With AI-powered voice transcriptions, technicians can simply record a voice memo to automatically fill out the required fields and add any necessary context to the work order. AI algorithms will automatically decipher complex speech patterns and analyze the context of the conversation to create precise transcriptions of the recording. Specially trained AI models can go a step further and accurately transcribe specialized terminology and jargon.
Improved communication and information sharing help maintenance teams identify potential failures faster, reduce the time it takes to fix breakdowns, and encourage collaboration among team members. This greatly increases the chances that there will be a complete record of the maintenance performed on each asset attached to a work order.
Barriers to Adopting Artificial Intelligence
Poor Data Quality
Garbage in, garbage out. AI models rely heavily on data to make accurate predictions and informed recommendations. When the data used to train and deploy the AI algorithms is riddled with inaccuracies, errors, or inconsistencies, it undermines the reliability and effectiveness of the system. Inaccurate or incomplete data can lead to misleading insights, incorrect recommendations, and biased outcomes, impacting decision-makers' confidence in the data analysis. Such data also poses significant risks, as inaccurate predictions can result in poor maintenance decisions, regulatory non-compliance, and reduced equipment uptime. Furthermore, rectifying data quality issues often requires substantial time, effort, and resources, adding to the cost of AI adoption.
To combat poor data quality, maintenance teams should implement robust data management practices, providing frontline workers with standardized templates to record data easily. This involves conducting regular data reviews to identify and fix oddities such as duplicate records, missing values, outliers, and inconsistencies. Teams should also invest in data quality tools and software to automate data cleansing, validation, and enrichment processes. Most importantly, you should focus on employee training and awareness to ensure that all team members understand the importance of data quality and their roles in maintaining it. By addressing data quality systematically, maintenance teams can unlock the full potential of AI, make more accurate decisions, and build trust in AI-driven solutions.
Decentralized Data
Decentralized data, siloed across various departments, locations, and formats, present a substantial hurdle to the widespread adoption of artificial intelligence. AI models require large volumes of high-quality data to train, and when that data is inaccessible, incomplete, or inconsistent, it impacts the models' effectiveness. Siloed data can easily lead to data duplication, making it challenging to maintain data quality and consistency across the organization. This challenge is particularly significant in larger organizations where data is generated and stored in diverse formats and locations.
To overcome the challenges of decentralized data, maintenance teams should look to consolidate data from various sources into a centralized repository like a CMMS or data warehouse, where it can be standardized and made accessible for training and analysis. Teams should also invest in robust data integration tools or platforms that provide seamless integrations out of the box to ensure easy access and simplify the transition from silos to a centralized repository.
Integrating Legacy Systems
Many maintenance teams operate with existing legacy systems that are not easily compatible with AI technologies. The problem is exacerbated by these older systems typically housing critical asset data and processes that AI models require. The complexity of integrating legacy systems with AI technologies, the risk of system disruption, and the high costs related to ensuring a smooth transition may make teams apprehensive about introducing AI technologies into their tech stack.
If your teams operate on legacy systems, take a tiered approach to integrating AI into your operations. First, collaborate with the IT team and conduct a thorough system audit to understand the current state of the technologies in place. Identify strategic areas for modernization and integration to prioritize based on potential strategic value and fast return. This process may involve using middleware or API gateways to bridge the gap between AI systems and legacy applications.
Additionally, organizations may consider reengineering legacy components or gradually replacing them with more modern, flexible solutions as a long-term strategy. It's crucial to invest in a team with expertise in both AI and legacy systems to ensure that integration projects proceed smoothly. By taking these steps, companies can gradually introduce AI into their operations while minimizing disruptions to legacy systems, ultimately reaping the benefits of enhanced efficiency and competitiveness.
Lack of Resources
Finding the right resources, whether financial, technical, or human, to properly implement AI systems can be a substantial barrier to adoption. Implementing AI applications often requires significant financial investment upfront to procure the right hardware, software, and expertise. The shortage of qualified AI experts, coupled with the high demand, can result in fierce competition, inflating the costs of hiring AI professionals and making it difficult to secure the right talent to drive AI initiatives. Resource constraints can deter organizations from embracing AI, especially when faced with rising costs of capital and materials, budget constraints, and resource limitations.
To overcome resource constraints, you should look to collaborate with service providers offering AI capabilities out of the box. While these solutions may not be fully customizable, your team will be able to access the power of artificial intelligence without the need for substantial in-house resources. This approach will save you time and money and give you a headstart over competitors struggling to onboard AI-based solutions.
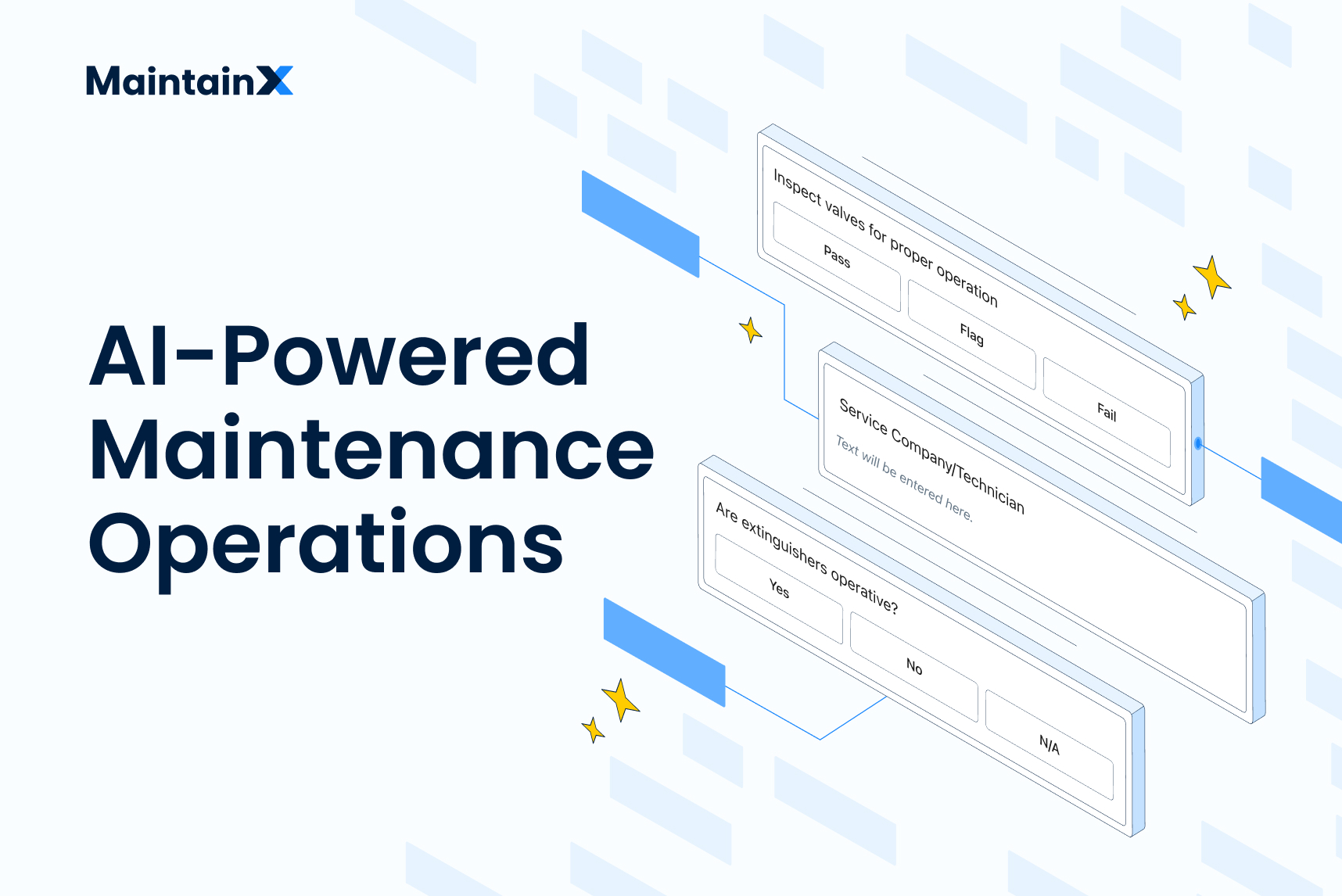
Optimize Your Maintenance Strategy with MaintainX AI Solutions
It’s rapidly becoming easier to take advantage of artificial intelligence to streamline work, scale productivity, and reduce downtime and waste. With out-of-the-box AI features like MaintainX’s Anomaly Detection, Voice Memos, and Procedure Generation, maintenance teams can easily reap the benefits of AI without needing to provide any data, training, or resources. Don’t fall behind. Book a tour of MaintainX to see how you can easily leverage AI technology to optimize your maintenance operations today.
Frequently Asked Questions
1. What is Artificial Intelligence? Artificial Intelligence (AI) is the science and engineering of intelligent machines or computer systems, i.e., systems that can mimic the problem-solving and decision-making capabilities of the human mind. AI also refers to machines or systems that display these traits.
2. What is GenAI (Generative Artificial Intelligence)? GenAI is a subset of artificial intelligence focused on creating systems that can generate content, such as text, images, or even music. This content is original and often indistinguishable from human-produced content. GenAI relies on deep learning models, particularly generative neural networks like GANs (Generative Adversarial Networks) and RNNs (Recurrent Neural Networks), which can produce data by learning patterns and structures from large datasets. Generative AI has various applications, including content generation, artistic creativity, language translation, and even deepfake generation, though it also raises ethical concerns related to misinformation and copyright issues.
3. What are the differences between AI and Machine Learning? Artificial Intelligence (AI) and Machine Learning (ML) are interconnected yet distinct domains in the field of computer science. AI encompasses the overarching concept of creating machines or software systems that replicate human-like intelligence, encompassing tasks such as problem-solving, decision-making, natural language understanding, and perception, using various techniques, including rule-based systems and expert systems, not all of which necessarily involve learning from data. On the other hand, Machine Learning is a subset of AI that specifically focuses on developing algorithms that learn from data, enabling systems to adapt and improve their performance over time without explicit programming for each task. ML excels in tasks requiring pattern recognition, prediction, and data-driven decision-making, making it an integral part of many AI applications.
4. How does AI improve itself? AI improves itself through a process known as "machine learning" and, more specifically, through "self-learning" or "reinforcement learning." In machine learning, AI systems are designed to learn from data and adapt their behavior based on patterns and feedback. They continuously analyze new data, identifying errors or areas where their performance can be enhanced.
FAQs
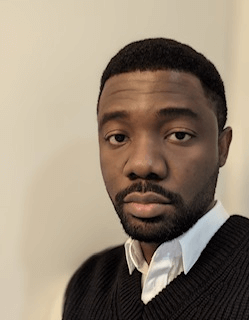
Lekan Olanrewaju
Lekan Olanrewaju is a content writer at MaintainX with years of experience in media and content creation. He has held positions at various media organizations, working with and leading teams at print magazines, digital publications, and television productions.